feeds
by zer0x0ne — on
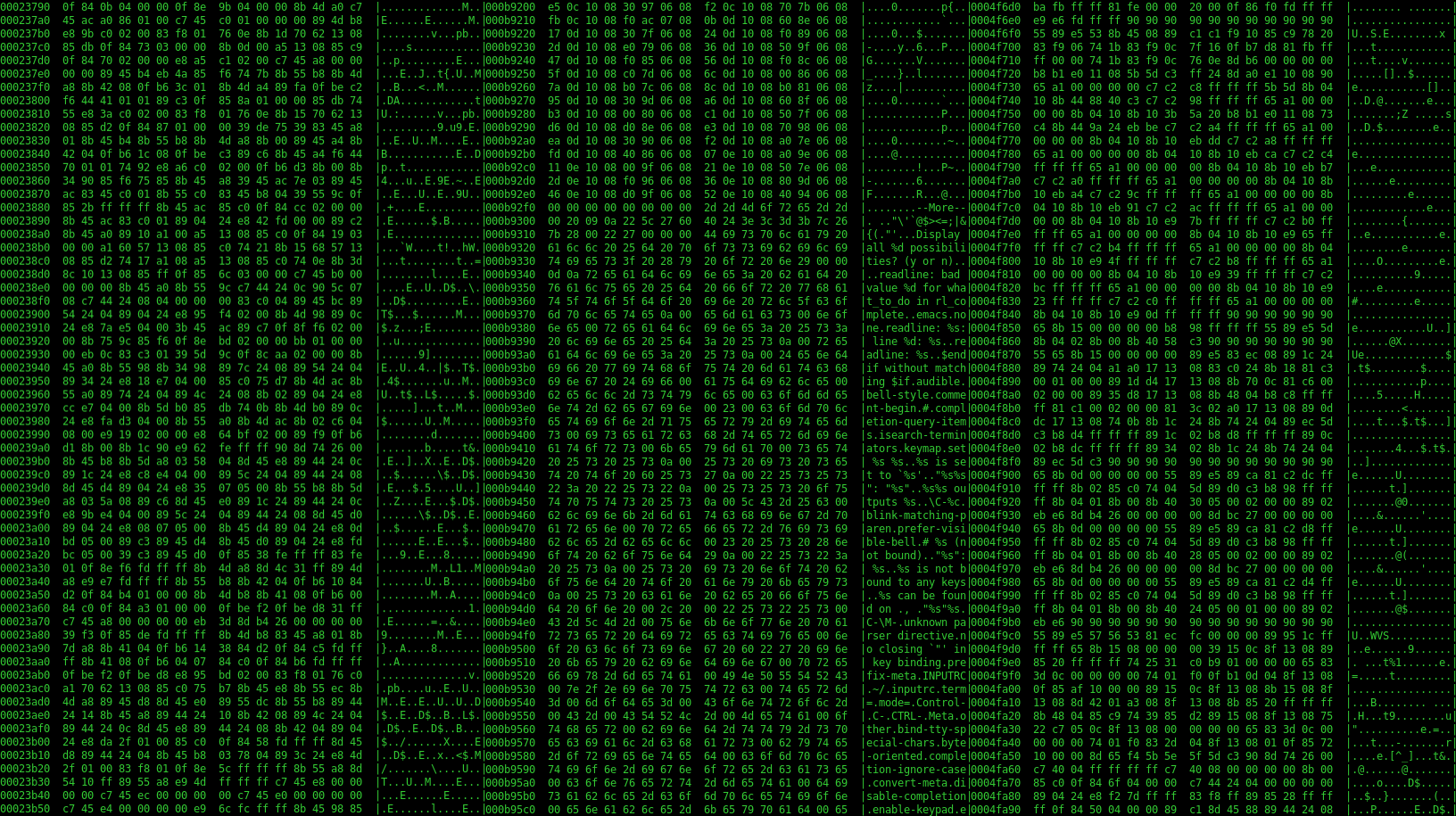
xkcd
Retrieved title: xkcd.com, 3 item(s)House Inputs and Outputs
President Venn Diagram
CrowdStrike
PortSwigger Research
Retrieved title: PortSwigger Research, 6 item(s)Fickle PDFs: exploiting browser rendering discrepancies
Imagine the CEO of a random company receives an email containing a PDF invoice file. In Safari and MacOS Preview, the total price displayed is £399. After approval, the invoice is sent to the accounti
A hacking hat-trick: previewing three PortSwigger Research publications coming to DEF CON & Black Hat USA
We're delighted to announce three major research releases from PortSwigger Research will be published at both Black Hat USA and DEF CON 32. In this post, we'll offer a quick teaser of each talk, info
onwebkitplaybacktargetavailabilitychanged?! New exotic events in the XSS cheat sheet
The power of our XSS cheat sheet is we get fantastic contributions from the web security community and this update is no exception. We had valuable contributions from Mozilla to remove events that no
Refining your HTTP perspective, with bambdas
When you open a HTTP request or response, what do you instinctively look for? Suspicious parameter names? CORS headers? Some clue as to the request's origin or underlying purpose? A single HTTP messag
Introducing SignSaboteur: forge signed web tokens with ease
Signed web tokens are widely used for stateless authentication and authorization throughout the web. The most popular format is JSON Web Tokens (JWT) which we've already covered in depth, but beyond t
Making desync attacks easy with TRACE
Have you ever found an HTTP desync vulnerability that seemed impossible to exploit due to its complicated constraints? In this blogpost we will explore a new exploitation technique that can be used to
Dark Reading
Retrieved title: darkreading, 6 item(s)Small Businesses Need Default Security in Products Now
Small businesses are increasingly being targeted by cyberattackers. Why, then, are security features priced at a premium?
Fighting Third-Party Risk With Threat Intelligence
With every new third-party provider and partner, an organization's attack surface grows. How, then, do enterprises use threat intelligence to enhance their third-party risk management efforts?
'Stargazer Goblin' Amasses Rogue GitHub Accounts to Spread Malware
The threat group uses its "Stargazers Ghost Network" to star, fork, and watch malicious repos to make them seem legitimate, all to distribute a variety of notorious information-stealers-as-a-service.
Cyberattackers Exploit Microsoft SmartScreen Bug in Stealer Campaign
The good news: Only organizations far behind on standard Windows patching have anything to worry about.
Hamster Kombat Players Threatened by Spyware & Infostealers
Players can only access the game by first joining its Telegram channel, with some going astray in copycat channels with hidden malware.
CrowdStrike Blames Crash on Buggy Security Content Update
CrowdStrike vows to provide customers with greater control over the delivery of future content updates by allowing granular selection of when and where these updates are deployed.
Almost Secure
Retrieved title: Almost Secure, 3 item(s)How insecure is Avast Secure Browser?
A while ago I already looked into Avast Secure Browser. Back then it didn’t end well for Avast: I found critical vulnerabilities allowing arbitrary websites to infect user’s computer. Worse yet: much of it was due to neglect of secure coding practices, existing security mechanisms were disabled for no good reason. I didn’t finish that investigation because I discovered that the browser was essentially spyware, collecting your browsing history and selling it via Avast’s Jumpshot subsidiary.
But that was almost five years ago. After an initial phase of denial, Avast decided to apologize and to wind down Jumpshot. It was certainly a mere coincidence that Avast was subsequently sold to NortonLifeLock, called Gen Digital today. Yes, Avast is truly reformed and paying for their crimes in Europe and the US. According to the European decision, Avast is still arguing despite better knowledge that their data collection was fully anonymized and completely privacy-conformant but… well, old habits are hard to get rid of.
Either way, it’s time to take a look at Avast Secure Browser again. Because… all right, because of the name. That was a truly ingenious idea to name their browser like that, nerd sniping security professionals into giving them free security audits. By now they certainly would have addressed the issues raised in my original article and made everything much more secure, right?

Note: This article does not present any actual security vulnerabilities. Instead, this is a high-level overview of design decisions that put users at risk, artificially inflating the attack surface and putting lots of trust into the many, many companies involved with the Avast webspaces. TL;DR: I wouldn’t run Avast Secure Browser on any real operating system, only inside a virtual machine containing no data whatsoever.
Contents
Summary of the findings
The issues raised in my original article about the pre-installed browser extensions are still partially present. Two extensions are relaxing the default protection provided by Content-Security-Policy even though it could have been easily avoided. One extension is requesting massive privileges, even though it doesn’t actually need them. At least they switched from jQuery to React, but they still somehow managed to end up with HTML injection vulnerabilities.
In addition, two extensions will accept messages from any Avast website – or servers pretending to be Avast websites, since HTTPS-encrypted connections aren’t being enforced. In the case of the Privacy Guard (sic!) extension, this messaging exposes users’ entire browsing information to websites willing to listen. Yes, Avast used to collect and sell that information in the past, and this issue could in principle allow them to do it again, this time in a less detectable way.
The Messaging extension is responsible for the rather invasive “onboarding” functionality of the browser, allowing an Avast web server to determine almost arbitrary rules to nag the user – or to redirect visited websites. Worse yet, access to internal browser APIs has been exposed to a number of Avast domains. Even if Avast (and all the other numerous companies involved in running these domains) are to be trusted, there is little reason to believe that such a huge attack surface can possibly be secure. So it has to be expected that other websites will also be able to abuse access to these APIs.
What is Avast Secure Browser?
Avast Secure Browser is something you get automatically if you don’t take care while installing your Avast antivirus product. Or AVG antivirus. Or Avira. Or Norton. Or CCleaner. All these brands belong to Gen Digital now, and all of them will push Avast Secure Browser under different names.
According to their web page, there are good reasons to promote this browser:

So one of the reasons is: this browser is 100% free. And it really is, as in: “you are the product.” I took the liberty of making a screenshot of the browser and marking the advertising space:

Yes, maybe this isn’t entirely fair. I’m still indecisive as to whether the search bar should also be marked. The default search engine is Bing and the browser will nudge you towards keeping it selected. Not because Microsoft’s search engine is so secure and private of course but because they are paying for it.
But these are quality ads and actually useful! Like that ad for a shop selling food supplements, so that you can lead a healthy life. A quick search reveals that one of the three food supplements shown in the ad is likely useless with the suspicion of being harmful. Another brings up lots of articles by interested parties claiming great scientifically proven benefits but no actual scientific research on the topic. Finally the third one could probably help a lot – if there were any way of getting it into your body in sufficient concentration, which seems completely impossible with oral intake.
Now that we got “free” covered, we can focus on the security and privacy aspects in the subsequent sections.
The pre-installed extensions
There are various reasons for browser vendors to pre-package extensions with their browser. Mozilla Firefox uses extensions to distribute experimental features before they become an integral part of the browser. As I learned back in 2011, Google Chrome uses such extensions to promote their web applications and give them an advantage over competition. And as Simon Willison discovered only a few days ago, the Google Hangouts extension built into Google Chrome gives Google domains access to internal browser APIs – quite nifty if one wants better user tracking capabilities.
My previous article mentioned Avast Secure Browser adding eleven extensions to the ones already built into Google Chrome. This number hasn’t changed: I still count eleven extensions, even though their purposes might have changed. At least that’s eleven extensions for me, differently branded versions of this browser seem to have a different combination of extensions. Only two of these extensions (Coupons and Video Downloader) are normally visible in the list of extensions and can be easily disabled. Three more extensions (Avast Bank Mode, Avast SecureLine VPN, Privacy Guard) become visible when Developer Mode is switched on.

And then there are five extensions that aren’t visible at all and cannot be disabled by regular means: Anti-Fingerprinting, Messaging, Side Panel, AI Chat, Phishing Protection. Finally, at least the New Tab extension is hardwired into the browser and is impossible to disable.
Now none of this is a concern if these extensions are designed carefully with security and privacy in mind. Are they?
Security mechanisms disabled
My previous article described the Video Downloader extension as a huge “please hack me” sign. Its extension manifest requested every permission possible, and it also weakened Content-Security-Policy (CSP) protection by allowing execution of dynamic scripts. Both were completely unnecessary, my proof of concept exploit abused it to get a foothold in the Avast Secure Browser.
Looking at the current Video Downloader manifest, things are somewhat better today:
{
"content_security_policy": "script-src 'self' 'unsafe-eval'; object-src 'self'",
"permissions": [
"activeTab", "downloads", "management", "storage", "tabs", "webRequest",
"webRequestBlocking", "<all_urls>"
],
}
The permissions requested by this extension still grant it almost arbitrary access to all websites. But at least the only unused privilege on this list is management
which gives it the ability to disable or uninstall other extensions.
As to CSP, there is still 'unsafe-eval'
which allowed this extension to be compromised last time. But now it’s there for a reason: Video Downloader “needs” to run some JavaScript code it receives from YouTube in order to extract some video metadata.
I did not test what this code is or what it does, but this grants at the very least the YouTube website the ability to compromise this extension and, via it, the integrity of the entire browser. But that’s YouTube, it won’t possibly turn evil, right?
For reference: it is not necessary to use 'unsafe-eval'
to run some untrusted code. It’s always possible to create an <iframe>
element and use the sandbox attribute to execute JavaScript code in it without affecting the rest of the extension.
But there are more extensions. There is the Avast Bank Mode extension for example, and its extension manifest says:
{
"content_security_policy": "script-src 'self' 'unsafe-eval'; object-src 'self'",
"permissions": [
"activeTab", "alarms", "bookmarks", "browsingData", "clipboardRead",
"clipboardWrite", "contentSettings", "contextMenus", "cookies", "debugger",
"declarativeContent", "downloads", "fontSettings", "geolocation", "history",
"identity", "idle", "management", "nativeMessaging", "notifications",
"pageCapture", "power", "privacy", "proxy", "sessions", "storage", "system.cpu",
"system.display", "system.memory", "system.storage", "tabCapture", "tabs", "tts",
"ttsEngine", "unlimitedStorage", "webNavigation", "webRequest",
"webRequestBlocking", "http://*/*", "https://*/*", "<all_urls>"
],
}
Yes, requesting every possible permission and allowing execution of dynamic scripts at the same time, the exact combination that wreaked havoc last time. Why this needs 'unsafe-eval'
? Because it uses some ancient webpack version that relies on calling eval()
in order to “load” JavaScript modules dynamically. Clearly, relaxing security mechanisms was easier than using a better module bundler (like the one used by other Avast extensions).
The (lack of) ad blocking privacy
The Privacy Guard extension is responsible for blocking ads and trackers. It is meant by the sentence “block ads and boost your online privacy” in the website screenshot above. It is also one of the two extensions containing the following entry in its manifest:
{
"externally_connectable": {
"ids": [ "*" ],
"matches": [
"*://*.avastbrowser.com/*",
"*://*.avgbrowser.com/*",
"*://*.ccleanerbrowser.com/*",
"*://*.avast.com/*",
"*://*.securebrowser.com/*"
]
},
}
What this means: any other extension installed is allowed to send messages to the Privacy Guard extension. That isn’t restricted to Avast extensions, any other extension you installed from Avast’s or Google’s add-on store is allowed to do this as well.
The same is true for any website under the domains avast.com
, securebrowser.com
, avastbrowser.com
, avgbrowser.com
or ccleanerbrowser.com
. Note that the rules here don’t enforce https://
scheme, unencrypted HTTP connections will be allowed as well. And while avast.com
domain seems to be protected by HTTP Strict Transport Security, the other domains are not.
Why this matters: when your browser requests example.securebrowser.com
website over an unencrypted HTTP connection, it cannot be guaranteed that your browser is actually talking to an Avast web server. In fact, any response is guaranteed to come from a malicious web server because to such website exists.
One way you might get a response from such a malicious web server is connecting to a public WiFi. In principle, anyone connected to the same WiFi could redirect unencrypted web requests to their own malicious web server, inject an invisible request to example.securebrowser.com
in a frame (which would also be handled by their malicious server) and gain the ability to message Privacy Guard extension. While not common, this kind of attack did happen in the wild.
And what does someone get then? Let me show you:
chrome.runtime.connect("onochehmbbbmkaffnheflmfpfjgppblm", {name: "PG_STORE"})
.onMessage.addListener(x => console.log(x));
This establishes a connection to the extension and logs all incoming messages. One message is received immediately:
{
"type": "chromex.state",
"payload": {
"main": {
"settings": {
"paused": false,
"off": false,
"blockingMode": "strict",
"showIconBadge": true,
"fingerprintEnabled": true,
"previousBlockingModeIsOff": false
},
"pausedDomains": [],
"whitelist": [],
"afpWhitelist": [],
"installationInfo": {
"hostPrefix": "",
"noProBrand": false,
"urls": {
"faqUrl": "https://extension.securebrowser.com/privacy-guard/learn-more/",
"proUrl": "https://extension.securebrowser.com/privacy-guard/offer/"
},
"whitelists": {
"whitelist": "https://update.avastbrowser.com/adblock/assets/v3/document_whitelist.txt",
"filterWhitelist": "https://update.avastbrowser.com/adblock/assets/v3/filter_whitelist.txt",
"searchWhitelist": "https://update.avastbrowser.com/adblock/assets/v3/search_document_whitelist.txt"
}
},
"isProUser": false,
"blockedAdsCount": 12
},
"tabs": {
"391731034": {
"adsBlocked": 0,
"fingerprintAttempts": 0,
"adsAllowed": 0,
"listAdsBlocked": [],
"listAdsAllowed": [],
"pageAllowed": false,
"isInternal": false,
"domainIsPaused": false,
"isInUserWhitelist": false,
"isInUserAfpWhitelist": false,
"netFilteringSwitch": true,
"active": true,
"audible": false,
"autoDiscardable": true,
"discarded": false,
"groupId": -1,
"height": 514,
"highlighted": true,
"id": 391731034,
"incognito": false,
"index": 2,
"lastAccessed": 1720641256405.484,
"mutedInfo": {
"muted": false
},
"openerTabId": 391731032,
"pendingUrl": "secure://newtab/",
"pinned": false,
"selected": true,
"status": "complete",
"title": "Example Domain",
"url": "https://example.com/",
"width": 299,
"windowId": 391730998,
"favIconUrl": "https://example.com/favicon.ico"
},
"-1": {
"adsBlocked": 0,
"fingerprintAttempts": 0,
"adsAllowed": 0,
"listAdsBlocked": [],
"listAdsAllowed": [],
"isInternal": true
},
"active": 391731034
}
}
}
The first part are the Privacy Guard settings, your whitelisted domains, everything. There are also the three hardcoded lists containing blocking exceptions – funny how Avast doesn’t seem to mention these anywhere in the user interface or documentation. I mean, it looks like in the default “Balanced Mode” their ad blocker won’t block any ads on Amazon or eBay among other things. Maybe Avast should be more transparent about that, or people might get the impression that this has something to do with those sponsored bookmarks.
And then there is information about all your browsing tabs which I shortened to only one tab here. It’s pretty much all information produced by the tabs API, enriched with some information on blocked ads. Privacy Guard will not merely send out the current state of your browsing session, it will also send out updates whenever something changes. To any browser extension, to any Avast website and to any web server posing as an Avast website.
Does Avast abuse this access to collect users’ browsing data again? It’s certainly possible. As long as they only do it for a selected subset of users, this would be very hard to detect however. It doesn’t help that Avast Secure Browser tracks virtual machine usage among other things, so it’s perfectly plausible that this kind of behavior won’t be enabled for people running one. It may also only be enabled for people who opened the browser a given number of times after installing it, since this is being tracked as well.
Can other browser extensions abuse this to collect users’ browsing data? Absolutely. An extension can declare minimal privileges, yet it will still be able to collect the entire browsing history thanks to Privacy Guard.
Can a malicious web server abuse this to collect users’ browsing data beyond a single snapshot of currently open tabs? That’s more complicated since this malicious web server would need its web page to stay open permanently somehow. While Avast has the capabilities to do that (more on that below), an arbitrary web server normally doesn’t and has to resort to social engineering.
The messaging interface doesn’t merely allow reading data, the data can also be modified almost arbitrarily as well. For example, it’s possible to enable ad blocking without any user interaction. Not that it changes much, the data collection is running whether ad blocking is enabled or not.
This messaging interface can also be used to add exceptions for arbitrary domains. And while Privacy Guard options page is built using React.js which is normally safe against HTML injections, in one component they chose to use a feature with the apt name dangerouslySetInnerHTML. And that component is used among other things for displaying, you guessed it: domain exceptions.
This is not a Cross-Site Scripting vulnerability, thanks to CSP protection not being relaxed here. But it allows injecting HTML content, for example CSS code to mess with Privacy Guard’s options page. This way an attacker could ensure that exceptions added cannot be removed any more. Or they could just make Privacy Guard options unusable altogether.
The onboarding experience
The other extension that can be messaged by any extension or Avast web server is called Messaging. Interestingly, Avast went as far as disabling Developer Tools for it, making it much harder to inspect its functionality. I don’t know why they did it, maybe they were afraid people would freak out when they saw the output it produces while they are browsing?

You wonder what is going on? This extension processes some rules that it downloaded from https://config.avast.securebrowser.com/engagement?content_type=messaging,messaging_prefs&browser_version=126.0.25496.127
(with some more tracking parameters added). Yes, there is a lot of info here, so let me pick out one entry and explain it:
{
"post_id": 108341,
"post_title": "[190] Switch to Bing provider – PROD; google",
"engagement_trigger_all": [
{
"parameters": [
{
"operator": "s_regex",
"value": "^secure:\\/\\/newtab",
"parameter": {
"post_id": 11974,
"name": "url_in_tab",
"post_title": "url_in_tab",
"type": "string"
}
}
]
},
{
"parameters": [
{
"operator": "s_regex",
"value": "google\\.com",
"parameter": {
"post_id": 25654,
"name": "setting_search_default",
"post_title": "setting_search_default (search provider)",
"type": "string"
}
}
]
}
],
"engagement_trigger_any": [
{
"parameters": [
{
"operator": "equals",
"value": "0",
"parameter": {
"post_id": 19236,
"name": "internal.triggerCount",
"post_title": "internal.triggerCount",
"type": "number"
}
}
]
},
{
"parameters": [
{
"operator": "n_gte",
"value": "2592000",
"parameter": {
"post_id": 31317,
"name": "functions.interval.internal.triggered_timestamp",
"post_title": "interval.internal.triggered_timestamp",
"type": "number"
}
}
]
}
],
"engagement_trigger_none": [],
…
}
The engagement_trigger_all
entry lists conditions that have all be true: you have to be on the New Tab page, and your search provider has to be Google. The engagement_trigger_any
entry lists conditions where any one is sufficient: this particular rule should not have been triggered before, or it should have been triggered more than 2592000 seconds (30 days) ago. Finally, engagement_trigger_none
lists conditions that should prevent this rule from applying. And if these conditions are met, the Messaging extension will inject a frame into the current tab to nag you about switching from Google to Bing:

Another rule will nag you every 30 days about enabling the Coupons extension, also a cash cow for Avast. There will be a nag to buy the PRO version for users opening a Private Browsing window. And there is more, depending on the parameters sent when downloading these rules probably much more.
An interesting aspect here is that these rules don’t need to limit themselves to information provided to them. They can also call any function of private Avast APIs under the chrome.avast
, chrome.avast.licensing
and chrome.avast.onboarding
namespaces. Some API functions which seem to be called in this way are pretty basic like isPrivateWindow()
or isConnectedToUnsafeWifi()
, while gatherInfo()
for example will produce a whole lot of information on bookmarks, other browsers and Windows shortcuts.
Also, displaying the message in a frame is only one possible “placement” here. The Messaging extension currently provides eight different user interface choices, including straight out redirecting the current page to an address provided in the rule. But don’t worry: Avast is unlikely to start redirecting your Google searches to Bing, this would raise too many suspicions.
Super-powered websites
Why is the Messaging extension allowing some Avast server to run browser APIs merely a side-note in my article? Thing is: this extension doesn’t really give this server anything that it couldn’t do all by itself. When it comes to Avast Secure Browser, Avast websites have massive privileges out of the box.
The browser grants these privileges to any web page under the avast.com
, avg.com
, avastbrowser.com
, avgbrowser.com
, ccleanerbrowser.com
and securebrowser.com
domains. At least here HTTPS connections are enforced, so that posing as an Avast website won’t be possible. But these websites automatically get access to:
- chrome.bookmarks API: full read/write access to bookmarks
- chrome.management API: complete access to extensions except for the ability to install them
chrome.webstorePrivate
API: a private browser API that allows installing extensions.- A selection of private Avast APIs:
chrome.avast
chrome.avast.licensing
chrome.avast.ntp
chrome.avast.onboarding
chrome.avast.ribbon
chrome.avast.safebrowsing
chrome.avast.safesearch
chrome.avast.stats
chrome.avast.themes
Now figuring out what all these private Avast APIs do in detail, what their abuse potential is and whether any of their crashes are exploitable requires more time than I had to spend on this project. I can see that chrome.avast.ntp
API allows manipulating the tiles displayed on the new tab page in arbitrary ways, including reverting all your changes so that you only see those sponsored links. chrome.avast.onboarding
API seems to allow manipulating the “engagement” data mentioned above, so that arbitrary content will be injected into tabs matching any given criteria. Various UI elements can be triggered at will. I’ll leave figuring out what else these can do to the readers. If you do this, please let me know whether chrome.avast.browserCall()
can merely be used to communicate with Avast’s Security & Privacy Center or exposes Chromium’s internal messaging.
But wait, this is Avast we are talking about! We all know that Avast is trustworthy. After all, they promised to the Federal Trade Commission that they won’t do anything bad any more. And as I said above, impersonating an Avast server won’t be possible thanks to HTTPS being enforced. Case closed, no issue here?
Not quite, there are far more parties involved here. Looking only at www.avast.com
, there is for example OneTrust who are responsible for the cookie banners. Google, Adobe, hotjar, qualtrics and mpulse are doing analytics (a.k.a. user tracking). A Trustpilot widget is also present. There is some number of web hosting providers involved (definitely Amazon, likely others as well) and at least two content delivery networks (Akamai and Cloudflare).
And that’s only one host. Looking further, there is a number of different websites hosted under these domains. Some are used in production, others are experiments, yet more appear to be abandoned in various states of brokenness. Some of these web services seem to be run by Avast while others are clearly run by third parties. There is for some reason a broken web shop run by a German e-commerce company, same that used to power Avira’s web shop before Gen Digital bought them.
If one were to count it all together, I would expect that a high two digit number of companies can put content on the domains mentioned above. I wouldn’t be surprised however if that number even went into three digits. Every single one of these companies can potentially abuse internal APIs of the Avast Secure Browser, either because they decide to make some quick buck, are coerced into cooperation by their government or their networks simply get compromised.
And not just that. It isn’t necessary to permanently compromise one of these web services. A simple and very common Cross-Site Scripting vulnerability in any one of these web services would grant any website on the internet access to these APIs. Did Avast verify the security and integrity of each third-party service they decided to put under these domains? I very much doubt so.
It would appear that the official reason for providing these privileges to so many websites was aiding the onboarding experience mentioned above. Now one might wonder whether such a flexible and extensive onboarding process is really necessary. But regardless of that, the reasonable way of doing this is limiting the attack surface. If you need to grant privileges to web pages, you grant them to a single host name. You make sure that this single host name doesn’t run any more web services than it absolutely needs, and that these web services get a proper security review. And you add as many protection layers as possible, e.g. the Content-Security-Policy mechanism which is severely underused on Avast websites.
I’ll conclude by quoting the decision to penalize Avast for their GDPR violations:
At this point, the Appellate Authority considers it necessary to recall that the Charged Company provides software designed to protect the privacy of its users. As a professional in the information and cyber field, the Charged Company is thereby also expected to be extremely knowledgeable in the field of data protection.
Yeah, well…
Numerous vulnerabilities in Xunlei Accelerator application
Xunlei Accelerator (迅雷客户端) a.k.a. Xunlei Thunder by the China-based Xunlei Ltd. is a wildly popular application. According to the company’s annual report 51.1 million active users were counted in December 2022. The company’s Google Chrome extension 迅雷下载支持, while not mandatory for using the application, had 28 million users at the time of writing.
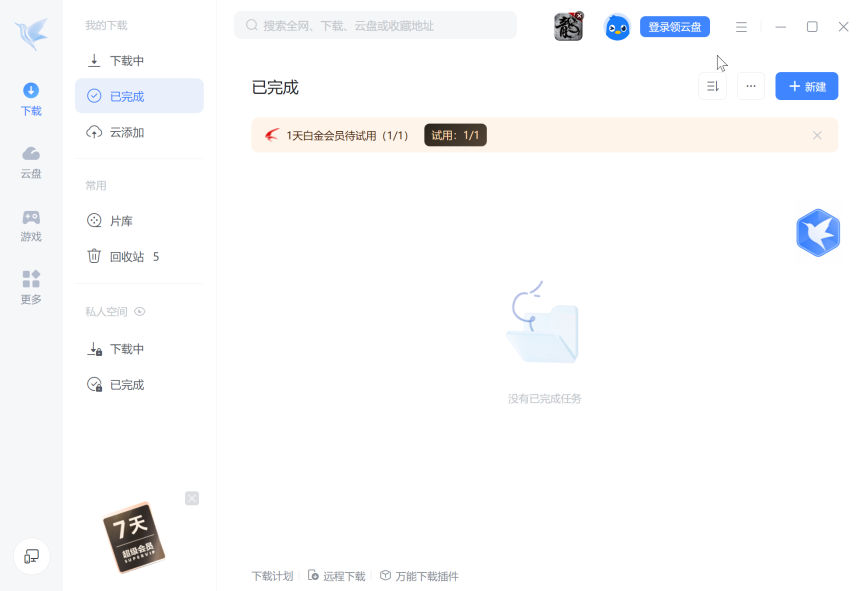
I’ve found this application to expose a massive attack surface. This attack surface is largely accessible to arbitrary websites that an application user happens to be visiting. Some of it can also be accessed from other computers in the same network or by attackers with the ability to intercept user’s network connections (Man-in-the-Middle attack).
It does not appear like security concerns were considered in the design of this application. Extensive internal interfaces were exposed without adequate protection. Some existing security mechanisms were disabled. The application also contains large amounts of third-party code which didn’t appear to receive any security updates whatsoever.
I’ve reported a number of vulnerabilities to Xunlei, most of which allowed remote code execution. Still, given the size of the attack surface it felt like I barely scratched the surface.
Last time Xunlei made security news, it was due to distributing a malicious software component. Back then it was an inside job, some employees turned rouge. However, the application’s flaws allowed the same effect to be easily achieved from any website a user of the application happened to be visiting.
Contents
What is Xunlei Accelerator?
Wikipedia lists Xunlei Limited’s main product as a Bittorrent client, and maybe a decade ago it really was. Today however it’s rather difficult to describe what this application does. Is it a download manager? A web browser? A cloud storage service? A multimedia client? A gaming platform? It appears to be all of these things and more.
It’s probably easier to think of Xunlei as an advertising platform. It’s an application with the goal of maximizing profits through displaying advertising and selling subscriptions. As such, it needs to keep the users on the platform for as long as possible. That’s why it tries to implement every piece of functionality the user might need, while not being particularly good at any of it of course.
So there is a classic download manager that will hijack downloads initiated in the browser, with the promise of speeding them up. There is also a rudimentary web browser (two distinctly different web browsers in fact) so that you don’t need to go back to your regular web browser. You can play whatever you are downloading in the built-in media player, and you can upload it to the built-in storage. And did I mention games? Yes, there are games as well, just to keep you occupied.
Altogether this is a collection of numerous applications, built with a wide variety of different technologies, often implementing competing mechanisms for the same goal, yet trying hard to keep the outward appearance of a single application.
The built-in web browser
The trouble with custom Chromium-based browsers
Companies love bringing out their own web browsers. The reason is not that their browser is any better than the other 812 browsers already on the market. It’s rather that web browsers can monetize your searches (and, if you are less lucky, also your browsing history) which is a very profitable business.
Obviously, profits from that custom-made browser are higher if the company puts as little effort into maintenance as possible. So they take the open source Chromium, slap their branding on it, maybe also a few half-hearted features, and they call it a day.
Trouble is: a browser has a massive attack surface which is exposed to arbitrary web pages (and ad networks) by definition. Companies like Mozilla or Google invest enormous resources into quickly plugging vulnerabilities and bringing out updates every six weeks. And that custom Chromium-based browser also needs updates every six weeks, or it will expose users to known (and often widely exploited) vulnerabilities.
Even merely keeping up with Chromium development is tough, which is why it almost never happens. In fact, when I looked at the unnamed web browser built into the Xunlei application (internal name: TBC), it was based on Chromium 83.0.4103.106. Being released in May 2020, this particular browser version was already three and a half years old at that point. For reference: Google fixed eight actively exploited zero-day vulnerabilities in Chromium in the year 2023 alone.
Among others, the browser turned out to be vulnerable to CVE-2021-38003. There is this article which explains how this vulnerability allows JavaScript code on any website to gain read/write access to raw memory. I could reproduce this issue in the Xunlei browser.
Protections disabled
It is hard to tell whether not having a pop-up blocker in this browser was a deliberate choice or merely a consequence of the browser being so basic. Either way, websites are free to open as many tabs as they like. Adding --autoplay-policy=no-user-gesture-required
command line flag definitely happened intentionally however, turning off video autoplay protections.
It’s also notable that Xunlei revives Flash Player in their browser. Flash Player support has been disabled in all browsers in December 2020, for various reasons including security. Xunlei didn’t merely decide to ignore this reasoning, they shipped Flash Player 29.0.0.140 (released in April 2018) with their browser. Adobe support website lists numerous Flash Player security fixes published after April 2018 and before end of support.
Censorship included
Interestingly, Xunlei browser won’t let users visit the example.com
website (as opposed to example.net
). When you try, the browser redirects you to a page on static.xbase.cloud
. This is an asynchronous process, so chances are good that you will catch a glimpse of the original page first.
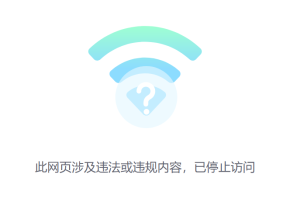
Automated translation of the text: “This webpage contains illegal or illegal content and access has been stopped.”
As it turns out, the application will send every website you visit to an endpoint on api-shoulei-ssl.xunlei.com
. That endpoint will either accept your choice of navigation target or instruct to redirect you to a different address. So when to navigate to example.com
the following request will be sent:
POST /xlppc.blacklist.api/v1/check HTTP/1.1
Content-Length: 29
Content-Type: application/json
Host: api-shoulei-ssl.xunlei.com
{"url":"http://example.com/"}
And the server responds with:
{
"code": 200,
"msg": "ok",
"data": {
"host": "example.com",
"redirect": "https://static.xbase.cloud/file/2rvk4e3gkdnl7u1kl0k/xappnotfound/#/unreach",
"result": "reject"
}
}
Interestingly, giving it the address http://example.com./
(note the trailing dot) will result in the response {"code":403,"msg":"params error","data":null}
. With the endpoint being unable to handle this address, the browser will allow you to visit it.
Native API
In an interesting twist, the Xunlei browser exposed window.native.CallNativeFunction()
method to all web pages. Calls would be forwarded to the main application where any plugin could register its native function handlers. When I checked, there were 179 such handlers registered, though that number might vary depending on the active plugins.
Among the functions exposed were ShellOpen
(used Windows shell APIs to open a file), QuerySqlite
(query database containing download tasks), SetProxy
(configure a proxy server to be used for all downloads) or GetRecentHistorys
(retrieve browsing history for the Xunlei browser).
My proof-of-concept exploit would run the following code:
native.CallNativeFunction("ShellOpen", "c:\\windows\\system32\\calc.exe");
This would open the Windows Calculator, just as you’d expect.
Now this API was never meant to be exposed to all websites but only to a selected few very “trusted” ones. The allowlist here is:
[
".xunlei.com",
"h5-pccloud.onethingpcs.com",
"h5-pccloud.test.onethingpcs.com",
"h5-pciaas.onethingpcs.com",
"h5-pccloud.onethingcloud.com",
"h5-pccloud-test.onethingcloud.com",
"h5-pcxl.hiveshared.com"
]
And here is how access was being validated:
function isUrlInDomains(url, allowlist, frameUrl) {
let result = false;
for (let index = 0; index < allowlist.length; ++index) {
if (url.includes(allowlist[index]) || frameUrl && frameUrl.includes(allowlist[index])) {
result = true;
break;
}
}
return result;
}
As you might have noticed, this doesn’t actually validate the host name against the list but looks for substring matches in the entire address. So https://malicious.com/?www.xunlei.com
is also considered a trusted address, allowing for a trivial circumvention of this “protection.”
Getting into the Xunlei browser
Now most users hopefully won’t use Xunlei for their regular browsing. These should be safe, right?
Unfortunately not, as there is a number of ways for webpages to open the Xunlei browser. The simplest way is using a special thunderx://
address. For example, thunderx://eyJvcHQiOiJ3ZWI6b3BlbiIsInBhcmFtcyI6eyJ1cmwiOiJodHRwczovL2V4YW1wbGUuY29tLyJ9fQ==
will open the Xunlei browser and load https://example.com/
into it. From the attacker’s point of view, this approach has a downside however: modern browsers ask the user for confirmation before letting external applications handle addresses.
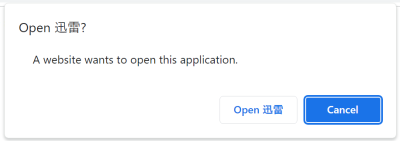
There are alternatives however. For example, the Xunlei browser extension (28 million users according to Chrome Web Store) is meant to pass on downloads to the Xunlei application. It could be instrumented into passing on thunderx://
links without any user interaction however, and these would immediately open arbitrary web pages in the Xunlei browser.
More ways to achieve this are exposed by the XLLite application’s API which is introduced later. And that’s likely not even the end of it.
The fixes
While Xunlei never communicated any resolution of these issues to me, as of Xunlei Accelerator 12.0.8.2392 (built on February 2, 2024 judging by executable signatures) several changes have been implemented. First of all, the application no longer packages Flash Player. It still activates Flash Player if it is installed on the user’s system, so some users will still be exposed. But chances are good that this Flash Player installation will at least be current (as much as software can be “current” three years after being discontinued).
The isUrlInDomains()
function has been rewritten, and the current logic appears reasonable. It will now only check the allowlist against the end of the hostname, matches elsewhere in the address won’t be accepted. So this now leaves “only” all of the xunlei.com domain with access to the application’s internal APIs. Any cross-site scripting vulnerability anywhere on this domain will again put users at risk.
The outdated Chromium base appears to remain unchanged. It still reports as Chromium 83.0.4103.106, and the exploit for CVE-2021-38003 still succeeds.
The browser extension 迅雷下载支持 also received an update, version 3.48 on January 3, 2024. According to automated translation, the changelog entry for this version reads: “Fixed some known issues.” The fix appears to be adding a bunch of checks for the event.isTrusted property, making sure that the extension can no longer be instrumented quite as easily. Given these restrictions, just opening the thunderx://
address directly likely has higher chances of success now, especially when combined with social engineering.
The main application
Outdated Electron framework
The main Xunlei application is based on the Electron framework. This means that its user interface is written in HTML and displayed via the Chromium web browser (renderer process). And here again it’s somewhat of a concern that the Electron version used is 83.0.4103.122 (released in June 2020). It can be expected to share most of the security vulnerabilities with a similarly old Chromium browser.
Granted, an application like that should be less exposed than a web browser as it won’t just load any website. But it does work with remote websites, so vulnerabilities in the way it handles web content are an issue.
Cross-site scripting vulnerabilities
Being HTML-based, the Xunlei application is potentially vulnerable to cross-site scripting vulnerabilities. For most part, this is mitigrated by using the React framework. React doesn’t normally work with raw HTML code, so there is no potential for vulnerabilities here.
Well, normally. Unless dangerouslySetInnerHTML
property is being used, which you should normally avoid. But it appears that Xunlei developers used this property in a few places, and now they have code displaying messages like this:
$createElement("div", {
staticClass: "xly-dialog-prompt__text",
domProps: { innerHTML: this._s(this.options.content) }
})
If message content ever happens to be some malicious data, it could create HTML elements that will result in execution of arbitrary JavaScript code.
How would malicious data end up here? Easiest way would be via the browser. There is for example the MessageBoxConfirm
native function that could be called like this:
native.CallNativeFunction("MessageBoxConfirm", JSON.stringify({
title: "Hi",
content: `<img src="x" onerror="alert(location.href)">`,
type: "info",
okText: "Ok",
cancelVisible: false
}));
When executed on a “trusted” website in the Xunlei browser, this would make the main application display a message and, as a side-effect, run the JavaScript code alert(location.href)
.
Impact of executing arbitrary code in the renderer process
Electron normally sandboxes renderer processes, making certain that these have only limited privileges and vulnerabilities are harder to exploit. This security mechanism is active in the Xunlei application.
However, Xunlei developers at some point must have considered it rather limiting. After all, their user interface needed to perform lots of operations. And providing a restricted interface for each such operation was too much effort.
So they built a generic interface into the application. By means of messages like AR_BROWSER_REQUIRE
or AR_BROWSER_MEMBER_GET
, the renderer process can instruct the main (privileged) process of the application to do just about anything.
My proof-of-concept exploit successfully abused this interface by loading Electron’s shell module (not accessible to sandboxed renderers by regular means) and calling one of its methods. In other words, the Xunlei application managed to render this security boundary completely useless.
The (lack of) fixes
Looking at Xunlei Accelerator 12.0.8.2392, I could not recognize any improvements in this area. The application is still based on Electron 83.0.4103.122. The number of potential XSS vulnerabilities in the message rendering code didn’t change either.
It appears that Xunlei called it a day after making certain that triggering messages with arbitrary content became more difficult. I doubt that it is impossible however.
The XLLite application
Overview of the application
The XLLite application is one of the plugins running within the Xunlei framework. Given that I never created a Xunlei account to see this application in action, my understanding of its intended functionality is limited. Its purpose however appears to be integrating the Xunlei cloud storage into the main application.
As it cannot modify the main application’s user interface directly, it exposes its own user interface as a local web server, on a randomly chosen port between 10500 and 10599. That server essentially provides static files embedded in the application, all functionality is implemented in client-side JavaScript.
Privileged operations are provided by a separate local server running on port 21603. Some of the API calls exposed here are handled by the application directly, others are forwarded to the main application via yet another local server.
I originally got confused about how the web interface accesses the API server, with the latter failing to implement CORS correctly – OPTION
requests don’t get a correct response, so that only basic requests succeed. It appears that Xunlei developers didn’t manage to resolve this issue and instead resorted to proxying the API server on the user interface server. So any endpoints available on the API server are exposed by the user interface server as well, here correctly (but seemingly unnecessarily) using CORS to allow access from everywhere.
So the communication works like this: the Xunlei application loads http://127.0.0.1:105xx/
in a frame. The page then requests some API on its own port, e.g. http://127.0.0.1:105xx/device/now
. When handling the request, the XLLite application requests http://127.0.0.1:21603/device/now
internally. And the API server handler within the same process responds with the current timestamp.
This approach appears to make little sense. However, it’s my understanding that Xunlei also produces storage appliances which can be installed on the local network. Presumably, these appliances run identical code to expose an API server. This would also explain why the API server is exposed to the network rather than being a localhost-only server.
The “pan authentication”
With quite a few API calls having the potential to do serious damage or at the very least expose private information, these need to be protected somehow. As mentioned above, Xunlei developers chose not to use CORS to restrict access but rather decided to expose the API to all websites. Instead, they implemented their own “pan authentication” mechanism.
Their approach of generating authentication tokens was taking the current timestamp, concatenating it with a long static string (hardcoded in the application) and hashing the result with MD5. Such tokens would expire after 5 minutes, apparently an attempt to thwart replay attacks.
They even went as far as to perform time synchronization, making sure to correct for deviation between the current time as perceived by the web page (running on the user’s computer) and by the API server (running on the user’s computer). Again, this is something that probably makes sense if the API server can under some circumstances be running elsewhere on the network.
Needless to say that this “authentication” mechanism doesn’t provide any value beyond very basic obfuscation.
Achieving code execution via plugin installation
There are quite a few interesting API calls exposed here. For example, the device/v1/xllite/sign
endpoint would sign data with one out of three private RSA keys hardcoded in the application. I don’t know what this functionality is used for, but I sincerely hope that it’s as far away from security and privacy topics as somehow possible.
There is also the device/v1/call
endpoint which is yet another way to open a page in the Xunlei browser. Both OnThunderxOpt
and OpenNewTab
calls allow that, the former taking a thunderx://
address to be processed and the latter a raw page address to be opened in the browser.
It’s fairly obvious that the API exposes full access to the user’s cloud storage. I chose to focus my attention on the drive/v1/app/install
endpoint however, which looked like it could do even more damage. This endpoint in fact turned out to be a way to install binary plugins.
I couldn’t find any security mechanisms preventing malicious software to be installed this way, apart from the already mentioned useless “pan authentication.” However, I couldn’t find any actual plugins to use as an example. In the end I figured out that a plugin had to be packaged in an archive containing a manifest.yaml
file like the following:
ID: Exploit
Title: My exploit
Description: This is an exploit
Version: 1.0.0
System:
- OS: windows
ARCH: 386
Service:
ExecStart: Exploit.exe
ExecStop: Exploit.exe
The plugin would install successfully under Thunder\Profiles\XLLite\plugin\Exploit\1.0.1\Exploit
but the binary wouldn’t execute for some reason. Maybe there is a security mechanism that I missed, or maybe the plugin interface simply isn’t working yet.
Either way, I started thinking: what if instead of making XLLite run my “plugin” I would replace an existing binary? It’s easy enough to produce an archive with file paths like ..\..\..\oops.exe
. However, the Go package archiver used here has protection against such path traversal attacks.
The XLLite code deciding which folder to put the plugin into didn’t have any such protections on the other hand. The folder is determined by the ID
and Version
values of the plugin’s manifest. Messing with the former is inconvenient, it being present twice in the path. But setting the “version” to something like ..\..\..
achieved the desired results.
Two complications:
- The application to be replaced cannot be running or the Windows file locking mechanism will prevent it from being replaced.
- The plugin installation will only replace entire folders.
In the end, I chose to replace Xunlei’s media player for my proof of concept. This one usually won’t be running and it’s contained in a folder of its own. It’s also fairly easy to make Xunlei run the media player by using a thunderx://
link. Behold, installation and execution of a malicious application without any user interaction.
Remember that the API server is exposed to the local network, meaning that any devices on the network can also perform API calls. So this attack could not merely be executed from any website the user happened to be visiting, it could also be launched by someone on the same network, e.g. when the user is connected to a public WiFi.
The fixes
As of version 3.19.4 of the XLLite plugin (built January 25, 2024 according to its digital signature), the “pan authentication” method changed to use JSON Web Tokens. The authentication token is embedded within the main page of the user interface server. Without any CORS headers being produced for this page, the token cannot be extracted by other web pages.
It wasn’t immediately obvious what secret is being used to generate the token. However, authentication tokens aren’t invalidated if the Xunlei application is restarted. This indicates that the secret isn’t being randomly generated on application startup. The remaining possibilities are: a randomly generated secret stored somewhere on the system (okay) or an obfuscated hardcoded secret in the application (very bad).
While calls to other endpoints succeed after adjusting authentication, calls to the drive/v1/app/install
endpoint result in a “permission denied” response now. I did not investigate whether the endpoint has been disabled or some additional security mechanism has been added.
Plugin management
The oddities
XLLite’s plugin system is actually only one out of at least five completely different plugin management systems in the Xunlei application. One other is the main application’s plugin system, the XLLite application is installed as one such plugin. There are more, and XLLiveUpdateAgent.dll
is tasked with keeping them updated. It will download the list of plugins from an address like http://upgrade.xl9.xunlei.com/plugin?os=10.0.22000&pid=21&v=12.0.3.2240&lng=0804
and make sure that the appropriate plugins are installed.
Note the lack of TLS encryption here which is quite typical. Part of the issue appears to be that Xunlei decided to implement their own HTTP client for their downloads. In fact, they’ve implemented a number of different HTTP clients instead of using any of the options available via the Windows API for example. Some of these HTTP clients are so limited that they cannot even parse uncommon server responses, much less support TLS. Others support TLS but use their own list of CA certificates which happens to be Mozilla’s list from 2016 (yes, that’s almost eight years old).
Another common issue is that almost all these various update mechanisms run as part of the regular application process, meaning that they only have user’s privileges. How do they manage to write to the application directory then? Well, Xunlei solved this issue: they made the application directory writable with user’s privileges! Another security mechanism successfully dismantled. And there is a bonus: they can store application data in the same directory rather than resorting to per-user nonsense like AppData.
Altogether, you better don’t run Xunlei Accelerator on untrusted networks (meaning: any of them?). Anyone on your network or anyone who manages to insert themselves into the path between you and the Xunlei update server will be able to manipulate the server response. As a result, the application will install a malicious plugin without you even noticing anything.
You also better don’t run Xunlei Accelerator on a computer that you share with other people. Anyone on a shared computer will be able to add malicious components to the Xunlei application, so next time you run it your user account will be compromised.
Example scenario: XLServicePlatform
I decided to focus on XLServicePlatform because, unlike all the other plugin management systems, this one runs with system privileges. That’s because it’s a system service and any installed plugins will be loaded as dynamic libraries into this service process. Clearly, injecting a malicious plugin here would result in full system compromise.
The management service downloads the plugin configuration from http://plugin.pc.xunlei.com/config/XLServicePlatform_12.0.3.xml
. Yes, no TLS encryption here because the “HTTP client” in question isn’t capable of TLS. So anyone on the same WiFi network as you for example could redirect this request and give you a malicious response.
In fact, that HTTP client was rather badly written, and I found multiple Out-of-Bounds Read vulnerabilities despite not actively looking for them. It was fairly easy to crash the service with an unexpected response.
But it wasn’t just that. The XML response was parsed using libexpat 2.1.0. With that version being released more than ten years ago, there are numerous known vulnerabilities, including a number of critical remote code execution vulnerabilities.
I generally leave binary exploitation to other people however. Continuing with the high-level issues, a malicious plugin configuration will result in a DLL or EXE file being downloaded, yet it won’t run. There is a working security mechanism here: these files need a valid code signature issued to Shenzhen Thunder Networking Technologies Ltd.
But it still downloads. And there is our old friend: a path traversal vulnerability. Choosing the file name ..\XLBugReport.exe
for that plugin will overwrite the legitimate bug reporter used by the Xunlei service. And crashing the service with a malicious server response will then run this trojanized bug reporter, with system privileges.
My proof of concept exploit merely created a file in the C:\Windows
directory, just to demonstrate that it runs with sufficient privileges to do it. But we are talking about complete system compromise here.
The (lack of?) fixes
At the time of writing, XLServicePlatform still uses its own HTTP client to download plugins which still doesn’t implement TLS support. Server responses are still parsed using libexpat 2.1.0. Presumably, the Out-of-Bounds Read and Path Traversal vulnerabilities have been resolved but verifying that would take more time than I am willing to invest.
The application will still render its directory writable for all users. It will also produce a number of unencrypted HTTP requests, including some that are related to downloading application components.
Outdated components
I’ve already mentioned the browser being based on an outdated Chromium version, the main application being built on top of an outdated Electron platform and a ten years old XML library being widely used throughout the application. This isn’t by any means the end of it however. The application packages lots of third-party components, and the general approach appears to be that none of them are ever updated.
Take for example the media player XMP a.k.a. Thunder Video which is installed as part of the application and can be started via a thunderx://
address from any website. This is also an Electron-based application, but it’s based on an even older Electron 59.0.3071.115 (released in June 2017). The playback functionality seems to be based on the APlayer SDK which Xunlei provides for free for other applications to use.
Now you might know that media codecs are extremely complicated pieces of software that are known for disastrous security issues. That’s why web browsers are very careful about which media codecs they include. Yet APlayer SDK features media codecs that have been discontinued more than a decade ago as well as some so ancient that I cannot even figure out who developed them originally. There is FFmpeg 2021-06-30 (likely a snapshot around version 4.4.4), which has dozens of known vulnerabilities. There is libpng 1.0.56, which was released in July 2011 and is affected by seven known vulnerabilities. Last but not least, there is zlib 1.2.8-4 which was released in 2015 and is affected by at least two critical vulnerabilities. These are only some examples.
So there is a very real threat that Xunlei users might get compromised via a malicious media file, either because they were tricked into opening it with Xunlei’s video player, or because a website used one of several possible ways to open it automatically.
As of Xunlei Accelerator 12.0.8.2392, I could not notice any updates to these components.
Reporting the issues
Reporting security vulnerabilities is usually quite an adventure, and the language barrier doesn’t make it any better. So I was pleasantly surprised to discover XunLei Security Response Center that was even discoverable via an English-language search thanks to the site heading being translated.
Unfortunately, there was a roadblock: submitting a vulnerability is only possible after logging in via WeChat or QQ. While these social networks are immensely popular in China, creating an account from outside China proved close to impossible. I’ve spent way too much time on verifying that.
That’s when I took a closer look and discovered an email address listed on the page as fallback for people who are unable to log in. So I’ve sent altogether five vulnerability reports on 2023-12-06 and 2023-12-07. The number of reported vulnerabilities was actually higher because the reports typically combined multiple vulnerabilities. The reports mentioned 2024-03-06 as publication deadline.
I received a response a day later, on 2023-12-08:
Thank you very much for your vulnerability submission. XunLei Security Response Center has received your report. Once we have successfully reproduced the vulnerability, we will be in contact with you.
Just like most companies, they did not actually contact me again. I saw my proof of concept pages being accessed, so I assumed that the issues are being worked on and did not inquire further. Still, on 2024-02-10 I sent a reminder that the publication deadline was only a month away. I do this because in my experience companies will often “forget” about the deadline otherwise (more likely: they assume that I’m not being serious about it).
I received another laconic reply a week later which read:
XunLei Security Response Center has verified the vulnerabilities, but the vulnerabilities have not been fully repaired.
That was the end of the communication. I don’t really know what Xunlei considers fixed and what they still plan to do. Whatever I could tell about the fixes here has been pieced together from looking at the current software release and might not be entirely correct.
It does not appear that Xunlei released any further updates in the month after this communication. Given the nature of the application with its various plugin systems, I cannot be entirely certain however.
Implementing a “Share on Mastodon” button for a blog
I decided that I would make it easier for people to share my articles on social media, most importantly on Mastodon. However, my Hugo theme didn’t support showing a “Share on Mastodon” button yet. It wasn’t entirely trivial to add support either: unlike with centralized solutions like Facebook where a simple link is sufficient, here one would need to choose their home instance first.
As far as existing solutions go, the only reasonably sophisticated approach appears to be Share₂Fedi. It works nicely, privacy-wise one could do better however. So I ended up implementing my own solution while also generalizing that solution to support a variety of different Fediverse applications in addition to Mastodon.
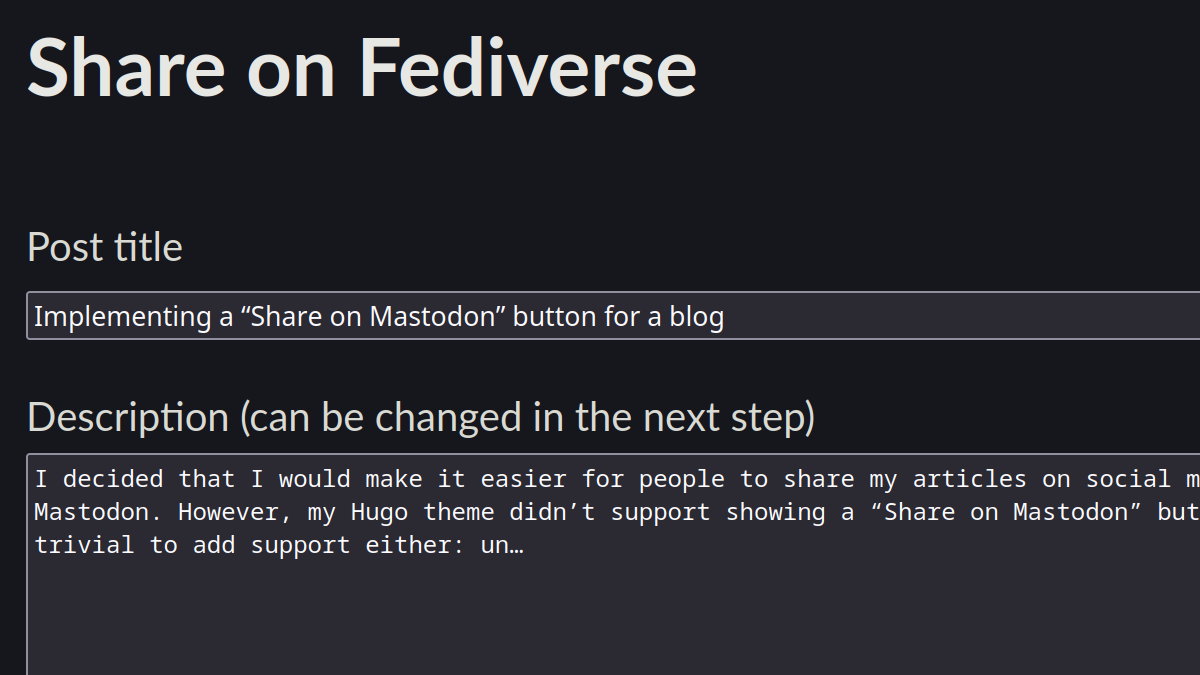
Contents
Why not Share₂Fedi?
If all you want is quickly adding a “Share on Fediverse” button, Share₂Fedi is really the simplest solution. It only requires a link, just like your typical share button. You link to the Share₂Fedi website, passing the text to be shared as a query parameter. The user will be shown an interstitial page there, allowing them to select a Fediverse instance. After submitting the form they will be redirected to the Fediverse instance in question for the final confirmation.
Unfortunately, the privacy aspect of this solution isn’t quite optimal. Rather than having all the processing happen on the client side, Share₂Fedi relies on server-side processing. This means that your data is being stored in the server logs at the very least. This data being the address and title of the article being shared, it isn’t terribly sensitive. Yet why send any data to a third party when you could send none?
I was told that Share₂Fedi was implemented in this way in order to work even with client-side JavaScript disabled. Which is a fair point but not terribly convincing seeing how your typical social media website won’t work without JavaScript.
But it is possible to self-host Share₂Fedi of course. It is merely something I’d rather avoid. See, this blog is built with the Hugo static site generator, and there is very little server-side functionality here. I’d rather keep it this way.
Share on Mastodon or on Fediverse?
Originally, I meant to add buttons for individual applications. First a “Share on Mastodon” button. Then maybe “Share on Lemmy.” Also “Share on Kbin.” Oh, maybe also Friendica. Wait, Misskey exposes the same sharing endpoint as Mastodon?
I realized that this approach doesn’t really scale, with the Fediverse consisting of many different applications. Supporting the applications beyond Mastodon is trivial, but adding individual buttons for each application would create a mess.
So maybe have a “Share on Fediverse” button instead of “Share on Mastodon”? Users have to select an instance anyway, and the right course of action can be determined based on the type of this instance. There is a Fediverse logo as well.
Only concern: few people know the Fediverse logo so far, way fewer than the people recognizing the Mastodon logo. So I decided to show both “Share on Mastodon” and “Share on Fediverse” buttons. When clicked, both lead to the exact same page.
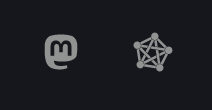
And that page would choose the right endpoint based on the selected instance. Here are the endpoints for individual Fediverse applications (mostly taken over from Share₂Fedi, some additions by me):
{
"calckey": "share?text={text}",
"diaspora": "bookmarklet?title={title}¬es={description}&url={url}",
"fedibird": "share?text={text}",
"firefish": "share?text={text}",
"foundkey": "share?text={text}",
"friendica": "compose?title={title}&body={description}%0A{url}",
"glitchcafe": "share?text={text}",
"gnusocial": "notice/new?status_textarea={text}",
"hometown": "share?text={text}",
"hubzilla": "rpost?title={title}&body={description}%0A{url}",
"kbin": "new/link?url={url}",
"mastodon": "share?text={text}",
"meisskey": "share?text={text}",
"microdotblog": "post?text=[{title}]({url})%0A%0A{description}",
"misskey": "share?text={text}"
}
Note: From what I can tell, Lemmy and Pleroma don’t have an endpoint which could be used.
What to share?
Share₂Fedi assumes that all Fediverse applications accept unstructured text. So that’s the default for my solution as well: a text consisting of the article’s title, description and address.
When it comes to the Fediverse, one size does not fit all however. Some applications like Diaspora expect more structured input. Micro.blog on the other hand expects Markdown input, special markup is required for a link to be displayed. And Kbin has the most exotic solution: it accepts only the article’s address, all other article metadata is then retrieved automatically.
So I resorted to displaying all the individual fields on the intermediate sharing page:
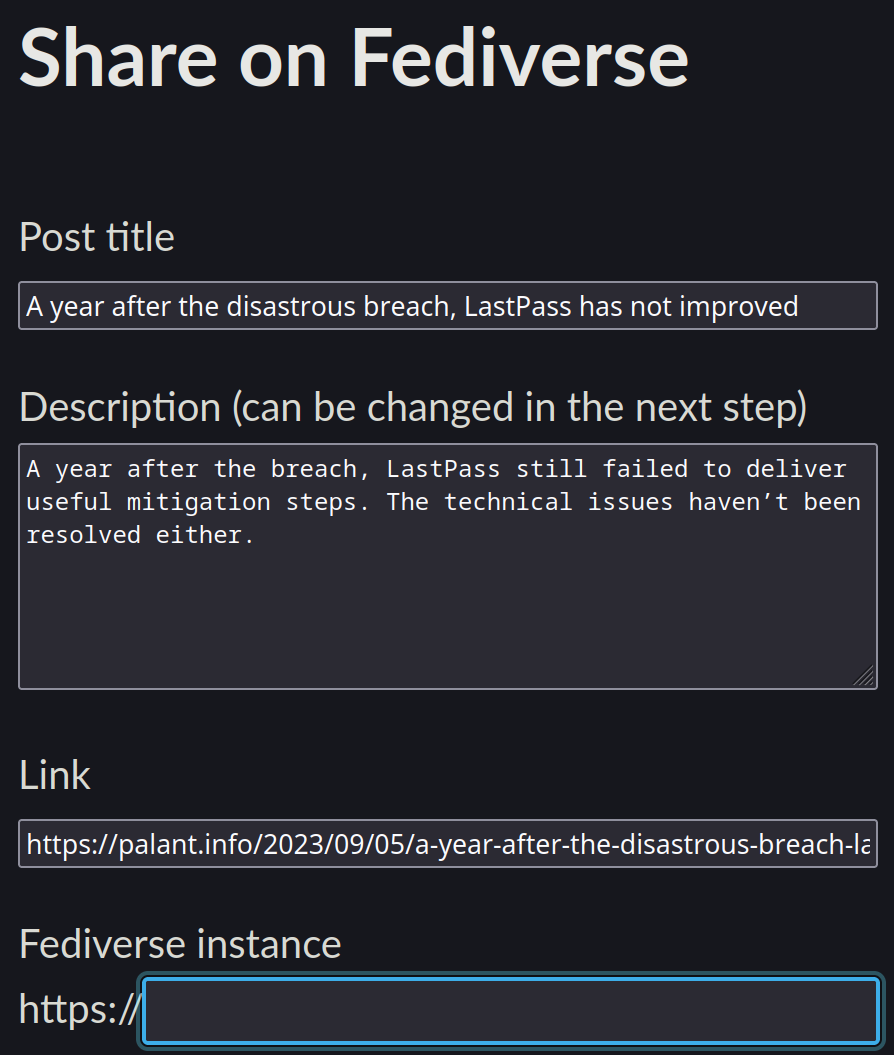
These fields are pre-filled and cannot be edited. After all, what good would editing these fields do if some of them might be thrown away or mashed together in the next step? So editing the text is delegated to the Fediverse instance, and this page is only about choosing an instance.
Trouble determining the Fediverse application
So, in order to choose the right endpoint, one has to know what Fediverse application powers the selected instance. Luckily, that’s easy. First, one downloads the .well-known/nodeinfo
file of the instance. Here is the one for infosec.exchange:
{
"links": [
{
"rel": "http://nodeinfo.diaspora.software/ns/schema/2.0",
"href": "https://infosec.exchange/nodeinfo/2.0"
}
]
}
We need the link marked with rel
value http://nodeinfo.diaspora.software/ns/schema/2.0
. Next we download this one and get:
{
"version": "2.0",
"software": {
"name": "mastodon",
"version": "4.3.0-alpha.0+glitch"
},
"protocols": ["activitypub"],
"services": {
"outbound": [],
"inbound": []
},
"usage": {
"users": {
"total": 60802,
"activeMonth": 17803,
"activeHalfyear": 33565
},
"localPosts": 2081420
},
"openRegistrations": true,
"metadata": {}
}
There it is: software name is identified as mastodon
, so we know to use the share?text=
endpoint.
The catch is: when I tried implementing this check, most Fediverse applications didn’t have consistent CORS headers on their node info responses. And this means that third-party websites (like my blog) would be allowed to request these endpoints but wouldn’t get access to the response. So no software name for me.
Now obviously it shouldn’t be like this, allowing third-party websites to access the node info is very much desirable. And most Fediverse applications being open source software, I fixed this issue for Mastodon, Diaspora, Friendica and Kbin. GNU Social, Misskey, Lemmy, Pleroma, Pixelfed and Peertube already had it working correctly.
But the issue remains: it will take quite some time until we can expect node info downloads to work reliably. One could use a CORS proxy of course, but it would run contrary to the goal of not relying on third parties. Or use a self-hosted CORS proxy, but that’s again adding server-side functionality.
I went with another solution. The Fediverse Observer website offers an API that allows querying its list of Fediverse instances. For example, the following query downloads information on all instances it knows.
{nodes(softwarename: ""){
softwarename
domain
score
active_users_monthly
}}
Unfortunately, it doesn’t have meaningful filtering capabilities. So I have to filter it after downloading: I only keep the servers with an uptime score above 90 and at least 10 active users in the past month. This results in a list of roughly 2200 instances, meaning 160 KiB uncompressed – a reasonable size IMHO, especially compared to the 5.5 MiB of the unfiltered list.
For my blog, Hugo will download this list when building the static site and incorporate it into the sharing page. So for most Fediverse instances, the page will already know what software is running on it. And if it doesn’t know an instance? Fall back to downloading the node info. And if that fails as well, just assume that it’s Mastodon.
Is this a perfect solution? Certainly not. Is it good enough? Yes, I think so. And we need that list of Fediverse instances anyway, for autocomplete functionality on the instance field.
The complete code
This solution is now part of the MemE theme for Hugo, see the corresponding commit. components/post-share.html
partial is where the buttons are being displayed. These link to the fedishare.html
page and pass various parameters via the anchor part of the URL (not the query string so that these aren’t being saved to server logs).
The fedishare.html
page is stored under assets. That’s because having a template turned into a static page would otherwise not happen by default and require additional changes to the configuration file. But that asset loads the fedishare.html
partial where the actual logic is located.
Building that page involves querying the Fediverse Observer API and filtering the response. Websites that are built too frequently can set up Hugo’s cache to avoid hitting the network every time.
The resulting list is put into a <datalist>
element, used for autocomplete on the instance field. The same list is also being used by the getSoftwareName()
function in the fedishare.js
asset, the script powering the page. Fallback for unknown instances is retrieving node info, and fallback here is just assuming Mastodon.
Once this chooses some Fediverse application, the script will take the corresponding endpoint, replace the placeholders by actual values and trigger navigation to that address.